Time series classification for dropout prediction#
We adopt the time series classification methodology presented in the work of Liu et al. [HWBT18] for student dropout prediction.
Time-series classifiers can effectively visualize and categorize data based on pattern similarities, enabling them to learn distinctive series of behaviors, distinguishing dropout from retention.
The classification approach uses student interaction data (click-stream) on different resource types (‘resource’, ‘content’ and ‘forumng’). Where ‘oucontent’ and ‘resource’ refer to a lecture video and a segment of text students are supposed to watch or read, and ‘forumng’ points to the forum space of the course.
A student is considered a dropout if he withdraws from a course.
In the OULAD dataset, this information is recorded with a Withdrawn
value in the
final_result
column of the student_info
table.
The authors rearranged the OULAD interaction data and transformed it into several time series using the following steps:
Extract the number of clicks the students make on the three types of material and group them by course module presentation.
Sum up the number of clicks each student makes on each type of material from each presentation daily.
Align each student’s total clicks on each type of material by days.
Add the dropout label, withdrawn as
1
, otherwise as0
, to the end of each student instance.
Thus, three time-series datasets are constructed for each course module presentation.
Finally, student dropout is predicted by applying a Time series forest classifier on each time series by course.
Liu Haiyang, Zhihai Wang, Phillip Benachour, and Philip Tubman. A time series classification method for behaviour-based dropout prediction. In 2018 IEEE 18th International Conference on Advanced Learning Technologies (ICALT), volume, 191–195. 2018. doi:10.1109/ICALT.2018.00052.
from typing import Iterator
import matplotlib.pyplot as plt
import numpy as np
import pandas as pd
from IPython.display import Markdown, display
from sklearn.model_selection import cross_val_score
from sktime.classification.interval_based import TimeSeriesForestClassifier
from oulad import get_oulad
%load_ext oulad.capture
%%capture oulad
oulad = get_oulad()
Dropout rate by course presentation#
As we embark on the task of student dropout classification, it’s important to first familiarize ourselves with some basic descriptive statistics about the dataset. As demonstrated in the work of Lui et al., a concise summary of domain information and relevant statistics regarding student dropout in diverse course modules can prove insightful. We shall attempt to replicate this table in our present study.
display(
# Add code_module, code_presentation, module_presentation_length columns.
oulad.courses
# Add domain column.
.merge(oulad.domains, on="code_module")
# Add days column.
.merge(
oulad.student_vle[oulad.student_vle.date < 0]
.groupby(["code_module", "code_presentation"])["date"]
.min()
.reset_index(),
on=["code_module", "code_presentation"],
)
.assign(days=lambda df: df.module_presentation_length - df.date + 1)
# Add id_student and final_result columns.
.merge(
oulad.student_info[
["id_student", "code_module", "code_presentation", "final_result"]
],
on=["code_module", "code_presentation"],
)
.assign(final_result=lambda df: df.final_result == "Withdrawn")
# Aggregate by course, rename final_result to dropout and id_student to students.
.groupby(["code_module", "code_presentation"])
.agg(
domain=("domain", "first"),
students=("id_student", "nunique"),
days=("days", "first"),
dropout=("final_result", "mean"),
)
# Format the dropout column using percentages.
.assign(dropout=lambda df: df.dropout.apply(lambda x: f"{x * 100:.2f}%"))
.reset_index()
)
code_module | code_presentation | domain | students | days | dropout | |
---|---|---|---|---|---|---|
0 | AAA | 2013J | Social Sciences | 383 | 279 | 15.67% |
1 | AAA | 2014J | Social Sciences | 365 | 294 | 18.08% |
2 | BBB | 2013B | Social Sciences | 1767 | 250 | 28.58% |
3 | BBB | 2013J | Social Sciences | 2237 | 292 | 28.79% |
4 | BBB | 2014B | Social Sciences | 1613 | 244 | 30.38% |
5 | BBB | 2014J | Social Sciences | 2292 | 272 | 32.68% |
6 | CCC | 2014B | STEM | 1936 | 260 | 46.38% |
7 | CCC | 2014J | STEM | 2498 | 288 | 43.11% |
8 | DDD | 2013B | STEM | 1303 | 257 | 33.15% |
9 | DDD | 2013J | STEM | 1938 | 280 | 35.14% |
10 | DDD | 2014B | STEM | 1228 | 260 | 39.90% |
11 | DDD | 2014J | STEM | 1803 | 288 | 35.88% |
12 | EEE | 2013J | STEM | 1052 | 280 | 23.10% |
13 | EEE | 2014B | STEM | 694 | 260 | 24.93% |
14 | EEE | 2014J | STEM | 1188 | 288 | 25.76% |
15 | FFF | 2013B | STEM | 1614 | 259 | 25.46% |
16 | FFF | 2013J | STEM | 2283 | 287 | 29.57% |
17 | FFF | 2014B | STEM | 1500 | 260 | 30.80% |
18 | FFF | 2014J | STEM | 2365 | 288 | 36.15% |
19 | GGG | 2013J | Social Sciences | 952 | 278 | 6.93% |
20 | GGG | 2014B | Social Sciences | 833 | 250 | 12.00% |
21 | GGG | 2014J | Social Sciences | 749 | 286 | 16.82% |
Prepare interaction data#
In this section, we attempt to replicate the interaction data transformations as described in the work of Liu et al.
%%capture -ns time_series_classification_for_dropout_prediction click_stream
click_stream = (
# 1. Extract the number of clicks by students on the three types of material.
oulad.vle.query("activity_type in ['resource', 'oucontent', 'forumng']")
.drop(["code_module", "code_presentation", "week_from", "week_to"], axis=1)
.merge(oulad.student_vle, on="id_site")
# 2. Sum the number of clicks each student makes on each type of material by day.
.groupby(
["code_module", "code_presentation", "id_student", "activity_type", "date"]
)
.agg({"sum_click": "sum"})
# 3. Align each student’s total clicks on each type of material by days.
.pivot_table(
values="sum_click",
index=["code_module", "code_presentation", "id_student", "activity_type"],
columns="date",
fill_value=0.0,
)
# 4. Add the dropout label, withdrawn as `1`, otherwise as `0`.
.join(
oulad.student_info.filter(
["code_module", "code_presentation", "id_student", "final_result"]
)
.assign(final_result=lambda df: (df.final_result == "Withdrawn").astype(int))
.set_index(["code_module", "code_presentation", "id_student"])
)
)
display(click_stream)
-25 | -24 | -23 | -22 | -21 | -20 | -19 | -18 | -17 | -16 | ... | 261 | 262 | 263 | 264 | 265 | 266 | 267 | 268 | 269 | final_result | ||||
---|---|---|---|---|---|---|---|---|---|---|---|---|---|---|---|---|---|---|---|---|---|---|---|---|
code_module | code_presentation | id_student | activity_type | |||||||||||||||||||||
AAA | 2013J | 11391 | forumng | 0.0 | 0.0 | 0.0 | 0.0 | 0.0 | 0.0 | 0.0 | 0.0 | 0.0 | 0.0 | ... | 0.0 | 0.0 | 0.0 | 0.0 | 0.0 | 0.0 | 0.0 | 0.0 | 0.0 | 0 |
oucontent | 0.0 | 0.0 | 0.0 | 0.0 | 0.0 | 0.0 | 0.0 | 0.0 | 0.0 | 0.0 | ... | 0.0 | 0.0 | 0.0 | 0.0 | 0.0 | 0.0 | 0.0 | 0.0 | 0.0 | 0 | |||
resource | 0.0 | 0.0 | 0.0 | 0.0 | 0.0 | 0.0 | 0.0 | 0.0 | 0.0 | 0.0 | ... | 0.0 | 0.0 | 0.0 | 0.0 | 0.0 | 0.0 | 0.0 | 0.0 | 0.0 | 0 | |||
28400 | forumng | 0.0 | 0.0 | 0.0 | 0.0 | 0.0 | 0.0 | 0.0 | 0.0 | 0.0 | 0.0 | ... | 0.0 | 0.0 | 0.0 | 0.0 | 0.0 | 0.0 | 0.0 | 0.0 | 0.0 | 0 | ||
oucontent | 0.0 | 0.0 | 0.0 | 0.0 | 0.0 | 0.0 | 0.0 | 0.0 | 0.0 | 0.0 | ... | 0.0 | 0.0 | 0.0 | 0.0 | 0.0 | 0.0 | 0.0 | 0.0 | 0.0 | 0 | |||
... | ... | ... | ... | ... | ... | ... | ... | ... | ... | ... | ... | ... | ... | ... | ... | ... | ... | ... | ... | ... | ... | ... | ... | ... |
GGG | 2014J | 2679821 | oucontent | 0.0 | 0.0 | 0.0 | 0.0 | 0.0 | 0.0 | 0.0 | 0.0 | 0.0 | 0.0 | ... | 0.0 | 0.0 | 0.0 | 0.0 | 0.0 | 0.0 | 0.0 | 0.0 | 0.0 | 1 |
resource | 0.0 | 0.0 | 0.0 | 0.0 | 0.0 | 0.0 | 0.0 | 0.0 | 0.0 | 0.0 | ... | 0.0 | 0.0 | 0.0 | 0.0 | 0.0 | 0.0 | 0.0 | 0.0 | 0.0 | 1 | |||
2684003 | forumng | 0.0 | 0.0 | 0.0 | 0.0 | 0.0 | 0.0 | 0.0 | 0.0 | 0.0 | 0.0 | ... | 0.0 | 0.0 | 0.0 | 0.0 | 0.0 | 0.0 | 0.0 | 0.0 | 0.0 | 0 | ||
oucontent | 0.0 | 0.0 | 0.0 | 0.0 | 0.0 | 0.0 | 0.0 | 0.0 | 0.0 | 0.0 | ... | 0.0 | 0.0 | 0.0 | 0.0 | 0.0 | 0.0 | 0.0 | 0.0 | 0.0 | 0 | |||
resource | 0.0 | 0.0 | 0.0 | 0.0 | 0.0 | 0.0 | 0.0 | 0.0 | 0.0 | 0.0 | ... | 0.0 | 0.0 | 0.0 | 0.0 | 0.0 | 0.0 | 0.0 | 0.0 | 0.0 | 0 |
80524 rows × 296 columns
Example#
Presented below is an extract of student interactions conducted within the “AAA_2013J” course presentation, specifically pertaining to forum activities. This extract corresponds to Table 2 as illustrated in the work of Lui et al.
display(
click_stream.loc[
[
("AAA", "2013J", 28400, "forumng"),
("AAA", "2013J", 30268, "forumng"),
("AAA", "2013J", 31604, "forumng"),
("AAA", "2013J", 2694424, "forumng"),
],
-10:"final_result",
]
)
-10 | -9 | -8 | -7 | -6 | -5 | -4 | -3 | -2 | -1 | ... | 261 | 262 | 263 | 264 | 265 | 266 | 267 | 268 | 269 | final_result | ||||
---|---|---|---|---|---|---|---|---|---|---|---|---|---|---|---|---|---|---|---|---|---|---|---|---|
code_module | code_presentation | id_student | activity_type | |||||||||||||||||||||
AAA | 2013J | 28400 | forumng | 14.0 | 0.0 | 4.0 | 17.0 | 8.0 | 3.0 | 4.0 | 0.0 | 23.0 | 0.0 | ... | 0.0 | 0.0 | 0.0 | 0.0 | 0.0 | 0.0 | 0.0 | 0.0 | 0.0 | 0 |
30268 | forumng | 5.0 | 0.0 | 2.0 | 0.0 | 0.0 | 0.0 | 0.0 | 9.0 | 17.0 | 6.0 | ... | 0.0 | 0.0 | 0.0 | 0.0 | 0.0 | 0.0 | 0.0 | 0.0 | 0.0 | 1 | ||
31604 | forumng | 0.0 | 6.0 | 0.0 | 0.0 | 18.0 | 0.0 | 0.0 | 0.0 | 5.0 | 0.0 | ... | 0.0 | 0.0 | 0.0 | 0.0 | 0.0 | 0.0 | 0.0 | 0.0 | 0.0 | 0 | ||
2694424 | forumng | 0.0 | 1.0 | 0.0 | 0.0 | 0.0 | 0.0 | 0.0 | 0.0 | 0.0 | 0.0 | ... | 0.0 | 0.0 | 0.0 | 0.0 | 0.0 | 0.0 | 0.0 | 0.0 | 0.0 | 0 |
4 rows × 281 columns
Dropout prediction by course#
Using the obtained click_stream
dataset, we train a Time series Forest
Classifier predicting students dropout for each course presentation.
As in the work of Lui et al. we set the number of trees in the Time series Forest to 500 and perform 10-fold cross-validation for each course module presentation using the classification accuracy as the evaluation metric.
%%capture -ns time_series_classification_for_dropout_prediction results
def get_scores_by_activity_type() -> Iterator[list[float]]:
"""Computes accuracy prediction scores for each course."""
for levels, course_df in click_stream.groupby(
level=["code_module", "code_presentation", "activity_type"]
):
estimator = TimeSeriesForestClassifier(n_estimators=500)
X = course_df.drop(columns="final_result").values
y = course_df["final_result"].values
mean_score = np.mean(
cross_val_score(estimator, X, y, cv=10, scoring="accuracy", n_jobs=-1)
)
yield list(levels) + [mean_score]
results = pd.DataFrame(
list(get_scores_by_activity_type()),
columns=["code_module", "code_presentation", "activity_type", "score"],
).pivot_table(
values="score",
index=["code_module", "code_presentation"],
columns="activity_type",
)
display(Markdown("### Dropout prediction 10-fold cross-validation accuracy by course"))
display(results)
Dropout prediction 10-fold cross-validation accuracy by course
activity_type | forumng | oucontent | resource | |
---|---|---|---|---|
code_module | code_presentation | |||
AAA | 2013J | 0.892817 | 0.936486 | 0.884282 |
2014J | 0.868908 | 0.892778 | 0.878235 | |
BBB | 2013B | 0.818326 | 0.779489 | 0.808844 |
2013J | 0.850292 | 0.874522 | 0.832293 | |
2014B | 0.843103 | 0.869054 | 0.846553 | |
2014J | 0.820690 | 0.843265 | 0.830800 | |
CCC | 2014B | 0.767816 | 0.743536 | 0.831991 |
2014J | 0.820959 | 0.778066 | 0.878427 | |
DDD | 2013B | 0.821852 | 0.786155 | 0.776506 |
2013J | 0.837887 | 0.835485 | 0.823526 | |
2014B | 0.778354 | 0.786464 | 0.807477 | |
2014J | 0.827989 | 0.871471 | 0.865274 | |
EEE | 2013J | 0.858427 | 0.843931 | 0.893902 |
2014B | 0.828415 | 0.821787 | 0.905333 | |
2014J | 0.884626 | 0.864434 | 0.918364 | |
FFF | 2013B | 0.835811 | 0.836155 | 0.853654 |
2013J | 0.821763 | 0.826214 | 0.825844 | |
2014B | 0.786010 | 0.816678 | 0.810618 | |
2014J | 0.852764 | 0.894428 | 0.874186 | |
GGG | 2013J | 0.959622 | 0.943264 | 0.940909 |
2014B | 0.915254 | 0.910090 | 0.917649 | |
2014J | 0.878409 | 0.886251 | 0.850469 |
Early dropout prediction#
The earlier we can accurately forecast student dropout, the more beneficial the approach becomes by affording MOOC instructors extra time for intervention. As in the work of Lui et al., we compare the predictive accuracy of the TimeSeriesForest classification using incrementally larger fractions of the original data. We start with a 5 percent dataset and iteratively add 5 percent increments to assess how prediction accuracy evolves.
%%capture -ns time_series_classification_for_dropout_prediction a_scores c_scores
def get_score_by_slice(index_slice) -> Iterator[float]:
"""Computes accuracy prediction scores for the given `index_slice`."""
y = click_stream.loc[index_slice, "final_result"].values
for i in range(5, 100, 5):
estimator = TimeSeriesForestClassifier(n_estimators=500)
limit = round((click_stream.columns.shape[0] - 1) * i / 100)
X = click_stream.loc[index_slice, click_stream.columns[:limit]].values
yield np.mean(
cross_val_score(estimator, X, y, cv=10, scoring="accuracy", n_jobs=-1)
)
a_scores = pd.Series(
list(get_score_by_slice(("AAA", "2013J", slice(None), "oucontent"))),
index=range(5, 100, 5),
)
c_scores = pd.Series(
list(get_score_by_slice(("CCC", "2014J", slice(None), "resource"))),
index=range(5, 100, 5),
)
for scores, name in [(a_scores, "AAA2013Joucontent"), (c_scores, "CCC2014Jresource")]:
scores.plot(
title=f"Accuracy score by the percentage of data used for {name} course",
xlabel="Percentage of data used",
ylabel="Prediction accuracy",
xticks=range(5, 100, 5),
figsize=(10, 5),
grid=True,
marker="s",
)
plt.show()
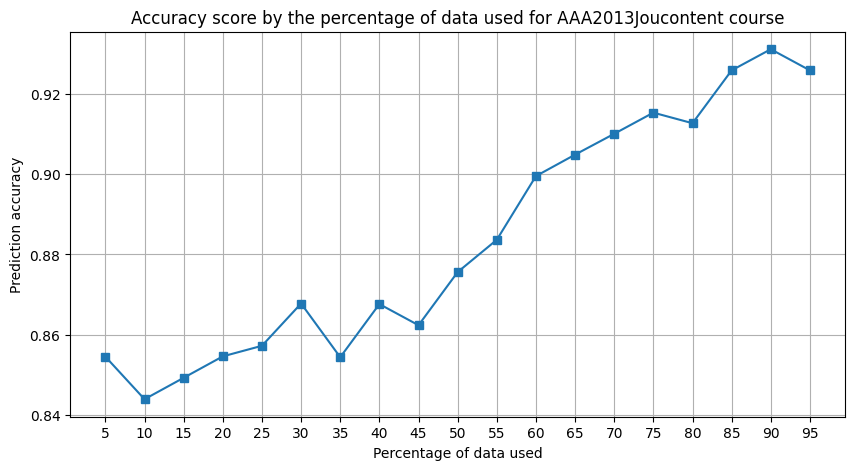
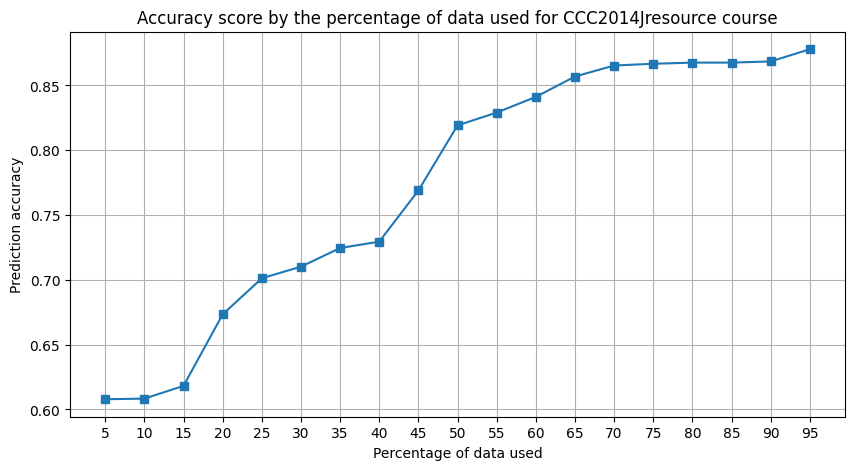